The Future of Data Integration: Trends & Technologies to Watch
- alexandragrundy
- 12 minutes ago
- 4 min read
Data is the heartbeat of modern business, but with an estimated 402.74 quintillion bytes created daily, making sense of it all is no small feat. Disconnected systems, inconsistent data streams, and siloed platforms mean organisations must act fast or risk falling behind.
To stay competitive, companies need to rethink their approach to data integration - connecting their data across platforms, in real time, with accuracy and security. The future of data integration is full of exciting possibilities, and those ready to embrace the latest technologies and trends stand to unlock significant opportunities.
Emerging Trends in Data Integration
Hybrid Data Integration
Hybrid integration is gaining traction as businesses increasingly combine on-premises and cloud-based systems. This approach offers organisations the flexibility to leverage the benefits of both environments - cloud scalability and on-premises control - while optimising performance and security. Hybrid integration minimises complexity by enabling businesses to manage data across multiple platforms and locations, whether on-premises, in the cloud, or at the edge. This method not only reduces latency by processing data closer to its source but also enhances data protection, compliance, and cost efficiency by minimising unnecessary data movement and optimising resource usage. As data volumes continue to grow, a hybrid model ensures organisations can effectively handle diverse data sources and meet evolving business and regulatory demands.
Real-Time Data Integration
The growing complexity and volume of data - along with the pressure to innovate and make agile decisions - is accelerating the adoption of real-time data integration technologies. Unlike traditional batch processing, these tools enable continuous data processing and analysis as information is generated, delivering immediate insights, reducing silos, and improving data quality. This capability is critical for real-time analytics and decision-making in high-stakes scenarios, such as fraud detection, hyper-personalised customer experiences, and AI-driven automation. Industries like finance, retail, and healthcare particularly benefit from this agility, where up-to-the-minute data is essential for maintaining a competitive edge.
Data Fabric
Unified data platforms like Microsoft Fabric are revolutionising data integration and analytics. Fabric brings together data lakes, warehouses, AI applications, and business intelligence capabilities, into a single, cohesive platform that supports the entire data lifecycle. Fabric reduces complexity by eliminating silos and promoting cross-functional collaboration. It provides a unified, real-time view of data across all sources, streamlining workflows and making data more accessible across departments. This holistic approach is reshaping how businesses handle data, driving both efficiency and innovation.
AI-Driven Data Integration
AI is revolutionising data integration by automating complex processes, enhancing data quality, and enabling real-time insights. Here's how AI is reshaping the data integration landscape:
Automating Pattern Recognition and Anomaly Detection: AI algorithms excel at identifying patterns and anomalies within vast datasets. By automating these tasks, AI reduces manual intervention, accelerates data processing, and uncovers insights that might be missed by traditional methods. This capability is crucial for businesses aiming to respond swiftly to changing data trends. For instance, machine learning algorithms can detect fraud in financial transactions by recognising unusual patterns.
Enhancing Data Quality and Consistency: Maintaining high data quality is essential for reliable analytics. AI aids in this by detecting inconsistencies, cleansing data, and ensuring uniformity across sources. Tools can help in identifying and rectifying duplicate or inconsistent records, thereby improving the overall integrity of the data. AI-driven data quality improvement processes can significantly reduce errors and enhance the reliability of insights.
Real-Time Data Processing and Feedback: The integration of AI enables real-time data analysis, allowing organisations to process and respond to information as it arrives. This immediacy is vital for applications such as fraud detection, customer feedback analysis, and dynamic decision-making. AI-driven tools can analyse streaming data, providing instant insights and facilitating prompt actions. For example, AI systems in e-commerce can adjust pricing dynamically based on real-time demand and inventory levels.
Predictive Analytics and Trend Forecasting: Beyond real-time analysis, AI empowers predictive analytics by forecasting future trends based on historical and current data. This foresight assists businesses in proactive planning and strategy development, ensuring they stay ahead in competitive markets. AI models can predict customer behaviour, helping companies tailor their marketing strategies effectively.
Streamlining Data Integration Workflows: AI simplifies the complexities of data integration by automating routine tasks, managing data pipelines, and ensuring seamless data flow between systems. This streamlining reduces the burden on IT teams and accelerates the deployment of data-driven solutions. AI-powered data mapping tools can automatically align schemas and transform data, reducing manual intervention and improving accuracy.
By leveraging AI in data integration, organisations can achieve more efficient, accurate, and responsive data management, paving the way for enhanced decision-making and innovation.
Best Practices for Modern Data Integration
1. Data Governance
Ensuring data quality and compliance is crucial in today’s regulatory environment. Implementing robust data governance frameworks is essential for maintaining data integrity, ensuring compliance with privacy regulations, and fostering trust in the insights derived from data. Effective governance strategies ensure that data is accurate, secure, and accessible when needed.
2. Scalability
As businesses continue to grow, so does the volume of their data. Modern data integration strategies must be scalable to handle these increasing demands. Cloud-based solutions and scalable architectures offer businesses the flexibility to scale their integration processes as needed, ensuring they can continue to meet the challenges of rapidly expanding data volumes.
3. Collaboration
Data integration shouldn’t be siloed - it should be a collaborative effort. Promoting cross-departmental collaboration ensures that all teams can access the data they need and share insights effectively. Encouraging collaboration between departments helps break down silos, streamline workflows, and unlock new opportunities for business optimisation and innovation.
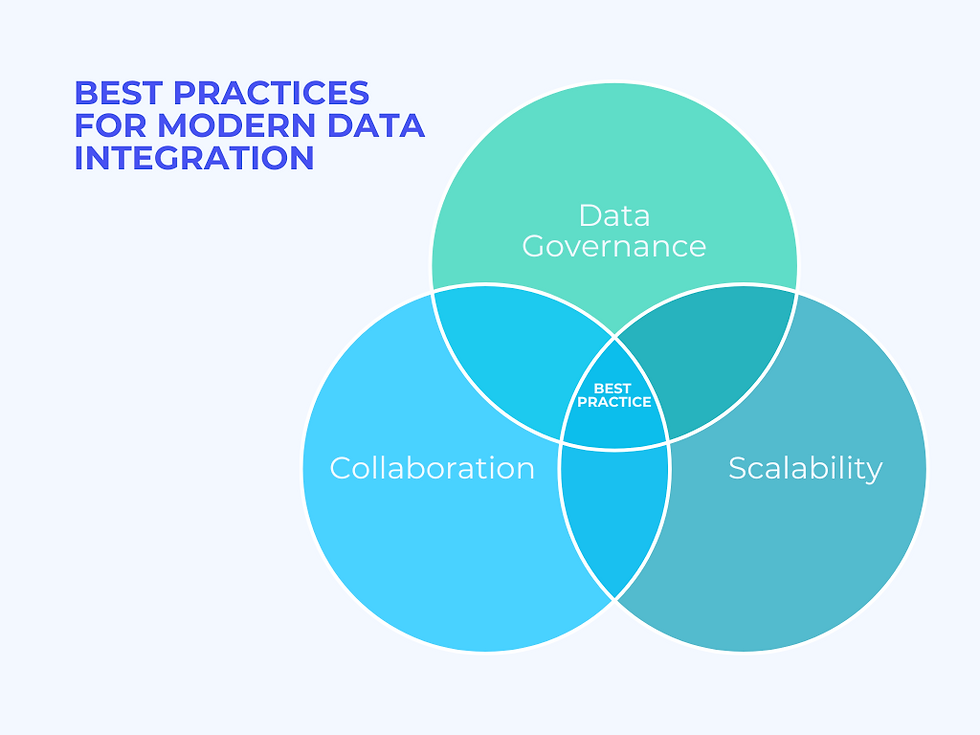
The future of data integration is bright, with emerging trends and cutting-edge technologies poised to transform the way businesses operate. By embracing hybrid integration, real-time data processing, and unified platforms like Microsoft Fabric, businesses can overcome traditional integration challenges and unlock new opportunities for growth, efficiency, and innovation.
The key to staying competitive in the data-driven world is to keep up with these trends and adopt technologies that enable smarter, faster, and more secure data integration.
Is your business ready to embrace the future of data integration? Don’t let outdated systems hold you back from leveraging the full potential of your data. Contact Solentive today to learn how we can help you implement modern data integration solutions, from AI-powered tools to unified platforms like Microsoft Fabric and stay ahead in the data-driven world.
Comments